AI in banking: key innovations and use cases you need to know
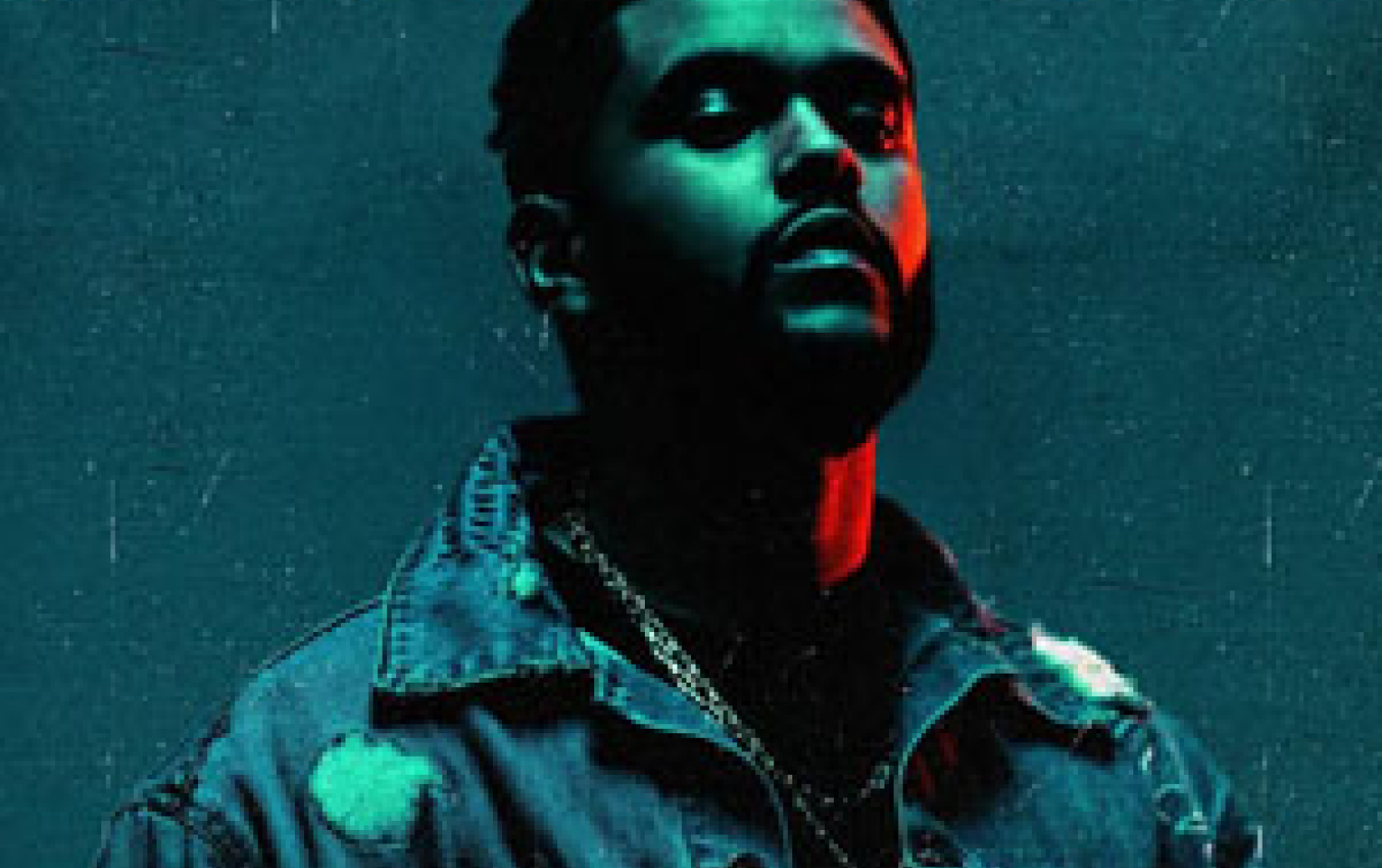
The banking industry is currently being totally rehauled with solutions brought by artificial intelligence banking. AI’s development in banking has evolved rapidly, helping banks streamline more processes, improve more security aspects, and deliver more personalized services.
As AI banking becomes essential for financial service providers and banks to keep pace with digital progress, it’s a must that we understand the technology’s most relevant applications. And today’s range of AI applications in banking stretches far beyond superficial and plainly routine process enhancements.
This article provides an in-depth look at the most impactful and promising AI in banking use cases, demonstrating its value in boosting efficiency, improving decision-making, and enhancing customer experience.
The role of AI in modern banking
AI and banking have turned into an inseparable match of today’s technology and service, allowing us to automate tasks, process vast amounts of data, and generate insights that help banks stay competitive. With AI-driven banking automation, providers can eliminate bottlenecks in routine processes, allowing human employees to focus on strategic activities.
- Automation of routine processes: Reduces human workload by automating repetitive tasks like data entry and transaction processing.
- Analytics-backed decision-making: AI in banking improves accuracy in financial forecasts and credit decisions, reducing human errors.
- Customer service AI in banking: With AI-based chatbots and virtual assistants for banking, banks get to provide faster, more downtime-free, and more reliable service.
Global banks like HSBC, JP Morgan, and Bank of America leverage AI to stay competitive by increasing efficiency, cutting operational costs, and better serving their customers. We’ll take a look at a bunch of major AI use cases in banking, so buckle up!
AI for fraud detection and prevention
Fraud detection is among the most relevant and forward-minded ways to employ AI in banking. AI-powered banking solutions can monitor each transaction taking place without downtime, automatically flagging suspicious activities and the tiniest indications of fraudulent behavior. Leading banks such as JP Morgan and HSBC use AI-driven fraud detection to minimize financial losses and protect their clients.
- Downtime-free monitoring: AI algorithms autonomously scan millions of transactions each second, picking out and reporting on any anomalies.
- Machine learning for risk prediction: Predicts potential fraud based on patterns and behavior, reducing risks before they escalate.
JP Morgan’s proprietary AI platform, “COiN” (Contract Intelligence), uses machine learning to analyze complex documents, such as legal contracts, for compliance and risk. This system has saved JP Morgan thousands of hours in document review and helped to significantly reduce human error.
AI in customer service (chatbots and virtual assistants)
AI in banks can power smart chatbots and virtual assistants, granting a proven way to smooth out and improve one’s customer service. Banks like Bank of America and Wells Fargo use intelligent banking chatbots to manage routine inquiries, providing clients with immediate responses.
- Personalized assistance: With Natural Language Processing (NLP), banking chatbots understand and respond to customer questions accurately.
- 24/7 availability: Chatbots help service providers and companies guarantee that customers get assistance anytime, cutting out waiting times and boosting post-service satisfaction.
Bank of America’s virtual assistant, “Erica,” supports customers by helping them check account balances, review transactions, and even make personalized financial recommendations.
HSBC’s chatbot, “Amy,” handles a range of customer service inquiries, reducing wait times and improving the customer experience. Amy is equipped with Natural Language Processing features, which allows her to understand customer intent and give lively, relevant answers. Inherently, she learns from previous user touchpoints to improve responses over time.
AI for credit scoring and risk assessment
AI reinvents your usual, cumbersome credit scoring by assessing creditworthiness with both traditional credit history and alternative behavioral data. With AI in credit scoring, banks make more informed lending decisions, cutting down on risks while expanding credit access to first-time and non-business users.
- Behavioral data analysis: AI evaluates spending habits, income, and payment history to paint a full picture of creditworthiness.
- Risk identification: AI for banking helps providers shape and offer personalized credit terms based on actual financial behaviors.
ZestFinance is known for pioneering the use of AI in credit scoring and risk assessment, making credit scoring more inclusive and lower on biases with their “ZAML” (Zest Automated Machine Learning) software, which includes features that detect and correct potential biases, empowering fairer lending practices.
AI in personalized financial services
AI enables banks to offer tailored financial advice, creating a more engaging customer experience. Mobile banking apps like Monzo and Revolut use AI to analyze spending and offer personalized financial guidance.
- Spending analysis: AI reviews transaction history and reports tendencies, projections, milestones, and recommendations for budgeting and saving.
- Investment recommendations: Based on spending and income, AI suggests personalized investment options to help customers grow wealth.
For one of the standout AI in banking examples, MoneyLion, a digital financial platform specialized in banking and financial advice, uses specialized banking AI to deliver custom advice based on user data, including personalized savings suggestions and spending insights.
Similarly, Nubank, a Brazilian fintech, uses AI in its mobile app to tailor credit options and provide financial recommendations, demonstrating that even mid-sized firms can leverage banking AI to double down on customer engagement and loyalty.
AI for predictive analytics in banking
AI-based predictive analytics in banking helps financial providers and banks understand customer behavior and anticipate market trends. By analyzing historical data, AI predicts future patterns, assisting banks with AI-driven risk management and investment strategies.
- Market trend prediction: Banks use AI to forecast rising or falling market prices, aiding in strategic decisions.
- Customer behavior forecasting: AI helps anticipate needs, enabling banks to offer timely and relevant financial products.
Medium-sized investment platforms like Betterment and Wealthfront use AI for banking to predict market conditions and recommend portfolio adjustments tailored to users’ investment goals. These AI-driven insights make sophisticated wealth management accessible to everyday investors, challenging the traditional exclusivity of large financial institutions.
AI in anti-money laundering (AML)
With all its autonomous pattern detection power, AI comes in more than handy in helping to prevent money laundering. Similarly to automated fraud detection, banks can use AI systems to track transactions and pinpoint any suspicious motions that could trace money laundering attempts. This approach helps banks comply with AML laws while efficiently monitoring vast amounts of transaction data.
- Automated suspicious activity detection: AI analyzes transaction patterns to flag high-risk transactions.
- Compliance monitoring: AI supports banks in adhering to AML regulations, such as those implemented by Danske Bank.
Danske Bank’s AI algorithms analyze transactions in real-time, continuously monitoring large volumes of data to spot unusual patterns. By building profiles based on normal transaction behavior, the AI can detect anomalies, such as unexpected transfers or transactions involving high-risk countries, and flag them for further investigation.
AI in process automation
Smart process automation is completely changing the way banks handle routine operations. By automating processes like transaction processing and loan applications, AI frees up resources and reduces errors. With repetitive tasks out of the way, AI saves banks time and costs, allowing employees to focus on value-added services.
- Loan application processing: AI reviews applications quickly, reducing approval times.
- Back-office automation: Banks like Deutsche Bank use smart automation to streamline document management and transaction processing.
AI-powered optical character recognition (OCR) technology helps Deutsche Bank automate document management by scanning, classifying, and extracting relevant information from financial documents, invoices, and contracts. The bank also uses intelligent bots to handle repetitive transaction processing tasks, such as reconciling accounts, processing payments, and managing trade confirmations.
AI in investment banking
AI solutions for banking have also become instrumental in investment banking. Mostly due to the ability to autonomously analyze extremely massive datasets and execute automated trading tasks. AI-based algorithms help banks make quick, data-driven investment decisions, minimizing underlying risk in the process.
- Automated trading systems: AI analyzes real-time market data to execute trades based on pre-set conditions.
- Big Data analysis: AI helps banks process huge amounts of unstructured data to find and leverage the most inviting investment opportunities.
Morgan Stanley uses AI and machine learning to assist its wealth management and investment banking divisions. The AI-powered platform Next Best Action analyzes market trends and client data to provide personalized investment recommendations for clients.
Challenges and limitations of AI in banking
While AI offers many benefits, it comes with challenges, such as data privacy, the need for extensive data for training, and integration into existing systems.
- Integration complexity: Legacy systems can be difficult to integrate with AI, slowing down implementation.
- Data dependency: AI requires large datasets for accurate predictions, which can be a barrier for some banks.
- Data protection and privacy: Customer/user data protection is unquestionably the highest-priority aspect, and your mechanisms must stay in line with regulations like the following.
GDPR
The General Data Protection Regulation applies if your implemented AI processes any personal data of EU-based individuals, which promotes lawfulness, fairness, integrity, transparency, and confidentiality of user data (Data Protection Impact Assessment is conducted in order to root out data risks and stick with the GDPR).
EU AI Act
The proposed Regulation on Artificial Intelligence is a risk-based regulatory framework that has only been gaining traction in the area of regulating AI in banking, but there’s no time like the present to start adapting AI principles to its forward-minded classifications (AIs are classified as unacceptable, high-risk, and limited-risk solutions, outlining prohibited practices which you must avoid to keep things fair, unbiased, and transparent, like manipulative AI and vulnerability exploitation.)
More EU regulations that might or might not affect you
PSD2
The Revised Payment Services Directive promotes secure customer authentication (SCA) and data sharing (which can be relevant when implementing AI in payment services);
DORA
The Digital Operational Resilience Act obliges banks to manage risks involved with Information and Communications Technology (ICT) that they employ for a resilient AI.
EBA Guidelines
The European Banking Authority provides two sets of guidelines describing risk management principles for AI + ML solutions.
Even for smaller fintechs like Chime and Stash, implementing AI can pose challenges, particularly around data privacy and security. However, many fintechs are overcoming these barriers by adopting flexible cloud-based AI banking solutions and emphasizing transparency in data practices.
Bottom line: what to expect from AI in banking
Banking and AI are undeniably a power couple to reckon with, reshaping and boosting everything from customer service to fraud prevention. The latest fruits of artificial intelligence and banking practices come together to automate senseless routines, toughen software security, and make services ultimately personalized and insightful for their end consumers.
Investing in AI banking solutions today is essential for providers of any scale, with any relation to financial services, that want to maintain a unique selling point and competitive edge in an increasingly digital world. As technology advances, the role of AI in banking will only grow, further enhancing the way banks serve their clients and manage their operations.
Are you ready to introduce your take on the ever-convenient banking AI? We can help easily overcome all of the above-mentioned challenges, maximize the benefits, and help you painlessly implement and set up competitive AI in banks.
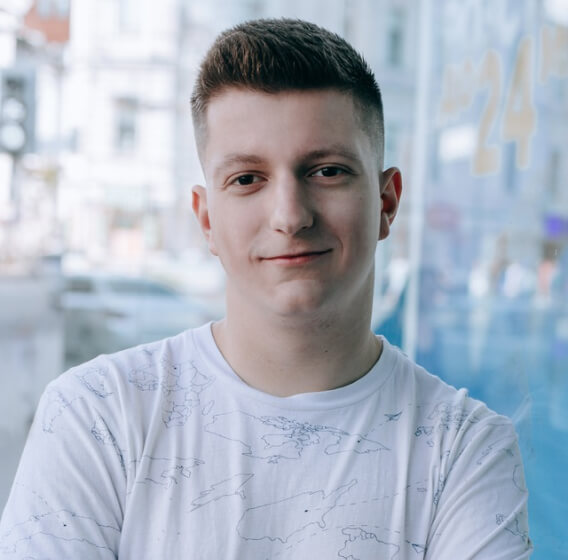
Similar articles
More about Product developmentStay updated with the latest case studies